by Sujit Raman and Thomas Armstrong
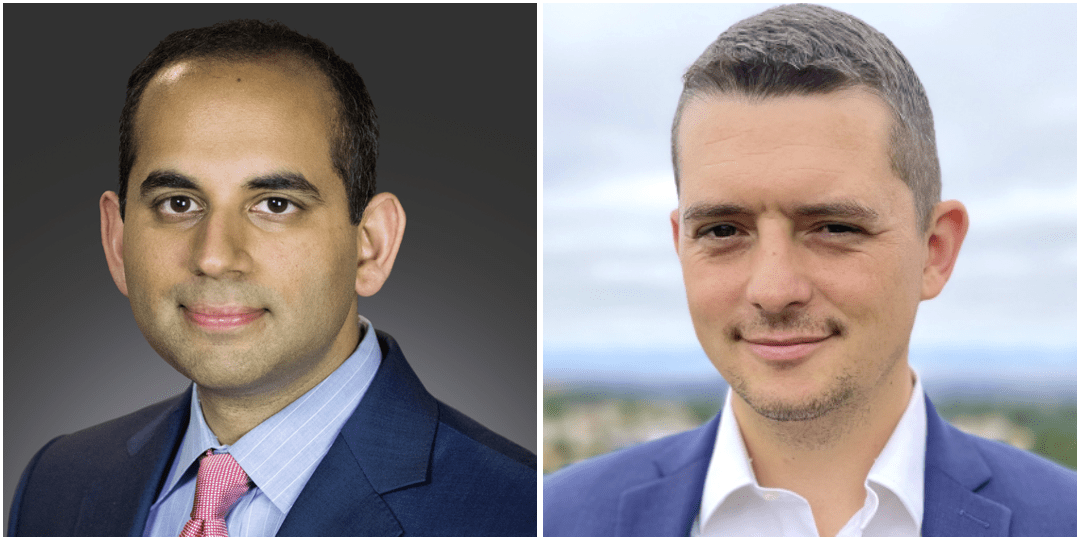
From left to right: Sujit Raman and Thomas Armstrong. (Photos courtesy of authors).
Everyone these days is talking about artificial intelligence and how to use it responsibly. Among law enforcement and compliance professionals, discussions around the responsible use of AI are nothing new. Even so, recent advances in machine learning have turbocharged AI’s transformative potential in detecting, preventing, and—in a particular sense—even predicting illicit activity. These advances are especially notable in the field of blockchain analytics: the process of associating digital asset wallets to real-world entities.
In a recent, pathbreaking opinion and order, U.S. District Judge Randolph Moss rejected a criminal defendant’s challenge to the government’s evidentiary use of blockchain analytics to link him to illicit financial activity.[1] Many courts—including, just a few days ago, a U.S. district court in Massachusetts[2]—have relied on the validity of blockchain analytics when taking pre-trial actions like issuing seizure orders and authorizing arrest warrants; Judge Moss’s opinion is the first trial court examination of this powerful analytic capability. Taken together, this growing body of legal authority forcefully affirms the reliability—and therefore admissibility in court—of evidence derived from such analytics.
While these rulings represent notable entries in the emerging caselaw around the evidentiary uses of AI, their significance isn’t merely doctrinal. Machine learning that leverages the analytic techniques endorsed in these rulings plays a vital role in mapping out the rapidly expanding digital infrastructure that serves as the backbone of the new Internet of Money. Understanding that infrastructure and mitigating the risks associated with it is critical to unlocking web 3’s virtually limitless potential.
Getting compliance “right” in this context also has immense practical importance. The total market capitalization of cryptocurrencies is resurging precisely when the illicit uses of digital assets are becoming more complex, and as the global security implications of the misuse of this technology become more pronounced. Unsurprisingly, State and federal regulators, as well as criminal enforcers, have vigorously entered the space. Compliance failures in the digital assets sector have led in recent months to the “largest settlements in [the U.S. Department of the Treasury’s] history,” the “largest corporate resolution to include criminal charges for an executive,” and the U.S. government’s “largest financial seizure ever,” among other headline-grabbing actions.
In other words, digital asset compliance matters. And while, according to the New York Department of Financial Services (NYDFS), the “unique characteristics” of virtual currencies—including the ability to transfer them “peer-to-peer directly from one individual or entity to another pseudonymously, absent the use of a regulated third party”—can “present compliance challenges,” those same characteristics “also present new possibilities for control measures that leverage these new technologies.” It is precisely the “native properties of blockchains—data that is transparent, traceable, public, permanent, private, and programmable”—that “enable[s] financial integrity professionals, law enforcement, regulators, supervisors, and other government agency officials to more readily identify risks and more effectively and efficiently detect and investigate financial crime.” Through blockchain analytics, this raw on-chain data can be converted into reliable, actionable intelligence.
What is blockchain analytics?
As noted above, blockchain analytics links a digital asset wallet address, which comprises of a randomly generated string of characters, to a real-world entity—such as a crypto exchange, a sanctioned actor, a mixing service, or a cybercriminal organization—with an appropriate level of confidence.
The actual linking process, known as “attribution,” can be achieved through a variety of means. It usually begins by leveraging open-source intelligence (e.g., wallet addresses publicly connected to identified entities or individuals via publication on OFAC’s SDN List) or by collecting threat intelligence (e.g., discovery by an intelligence expert of a wallet address that a ransomware organization is using to collect extortion payments, or that a terrorist organization is using to raise funds). These attribution techniques produce results with a high degree of confidence because they typically employ first-hand, primary source methods (e.g., direct interaction with the owner or user of the wallet).
Due to the way it is collected, however, attribution based on open-source or threat intelligence tends to be small-scale in scope. And yet, the number of blockchains, wallet addresses, and on-chain transactions is constantly growing, at rates that far exceed the capacity of primary attribution methods. Blockchain analytics firms address this challenge by taking high-confidence primary “ground truth” data and using machine learning[3] to achieve additional attribution at scale.
How does this work? At bottom, attribution at scale is a question of prediction, which, as the social scientists Ajay Agrawal, Joshua Gans, and Avi Goldfarb have observed, is “the process of filling in missing information” by “tak[ing] information you have . . . and us[ing] it to generate information you don’t have.” Human beings do this all the time; we employ what psychologists call “heuristics” to “navigat[e] day-to-day life” and “make countless small decisions within a limited timeframe,” constantly updating our decision-making frameworks based on the “ground truth” of lived experience. In computer science, “heuristics” play a similar role: in the face of massive amounts of data requiring analysis, they “produce a workable and practical solution . . . in a reasonable time,” “providing quick results with an acceptable accuracy range rather than offering near-perfect solutions.”
Data scientists can apply different heuristics to solve a big data problem. For example, a basic concept in machine learning is “clustering,” which involves grouping like examples together as a first step in understanding large data sets. Within blockchain analytics, “clustering tools rapidly scan the blockchain . . . to conduct various forms of pattern recognition.” Using different heuristics, one can cluster blockchain addresses together based on similar features, characteristics, and behaviors, and draw probabilistic conclusions about them—including about their ownership.
Judge Moss describes in his opinion the well-known “co-spend” or “common spend” heuristic, wherein a sender of a transaction draws on funds held in multiple addresses before transferring them to the receiver. Because each sending wallet address has a private key needed to initiate the transaction, it is highly likely that each such address is owned by the same entity and can therefore be clustered together.
Another heuristic may be “based on observing and tracking a particular entity’s on-chain behaviors and patterns.” For example, a cryptocurrency exchange may follow a standard (and therefore recognizable) set of internal procedures when receiving customer deposits and moving them into other wallets under the exchange’s control. Likewise, a ransomware organization may employ consistent (and therefore recognizable) techniques when laundering extortion proceeds. Building off high-quality primary intelligence, blockchain analytics firms use machine learning to identify distinctive (or anomalous) patterns through the noise of trillions of on-chain transactions and to fine-tune their heuristics—thereby automating and greatly enriching the attribution process.
This early example involving the use of machine learning to automatically identify and label one million wallets on the Ethereum blockchain belonging to crypto exchanges brings the above-described concepts to life. (In the intervening years, clustering methods and the heuristics associated with them have become only more complex. The same is true of on-chain obfuscation techniques.) Attribution projects of this sort have obvious relevance to the work of compliance professionals and law enforcement officers. Indeed, machine learning-enhanced attribution may be particularly important in criminal, regulatory, and compliance investigations involving large crypto services such as exchanges or mixers, as these services often use thousands if not millions of wallet addresses to manage transactional activity. (The ability to automatically detect such patterns using AI/ML may also become increasingly important in light of the recent FinCEN Notice of Proposed Rule Making that may institute a reporting and record-keeping requirement for any on-chain transactions that appear to obfuscate the source or destination of funds. Moreover, agencies including NYDFS and OFAC already have issued guidance and taken actions that emphasize the importance of using blockchain analytics tools in connection with industry compliance efforts under various State and federal laws.)
Conclusion
Blockchain analytics leverages the power of AI/ML to group relevant digital wallet addresses together, resulting in the faster and more effective tracing and tracking of funds in order to identify their origination or destination. As the use of these analytic capabilities grows, legal and compliance professionals will need to become familiar with the concepts that are central to their validity.
Judge Moss is the first judge to examine these capabilities and assess their reliability through a detailed, written opinion after receiving evidence and conducting adversarial hearings. His conclusion—that the output of blockchain intelligence tools can be “the product of reliable principles and methods” and that the analytic techniques upon which they rely may “assist the jury in understanding the overwhelming mass of data found on the blockchain,” see Daubert v. Merrell Dow Pharmaceuticals, Inc., 509 U.S. 579 (1993); Fed. R. Evid. 702—represents a watershed finding in the application of machine learning to financial crime compliance. That finding may well have significant follow-on implications as the usage of AI/ML models continues to expand across industries, and as machine learning analytics not only continue to reveal key evidence in crypto investigations, but also come to underpin banks’ transaction monitoring programs to detect suspicious activities and money laundering patterns in the traditional financial system, as well.
Footnotes
[1] Based in part on this evidence, a federal jury convicted the defendant of operating one of “the longest-running and most prolific bitcoin money laundering services on the darknet.” Press Release, U.S. Dep’t of Justice, “Jury Finds Russian-Swedish Operator of ‘Bitcoin Fog’ Guilty of Running the Darknet Cryptocurrency Mixer,” March 12, 2024.
[2] See Press Release, U.S. Dep’t of Justice, “United States Files Forfeiture Action to Recover Cryptocurrency Traceable to Pig Butchering Romance Scam,” March 13, 2024. We should note that this action involved investigators’ use of our firm’s platform; see TRM Labs, Insights, “DOJ, Secret Service Seek Forfeiture of $2.3 Million in Cryptocurrency Tied to Pig Butchering,” March 20, 2024 (observing that federal agents submitted visual graphs created through their use of TRM Labs software to support their application seeking court authorization to seize alleged fraud proceeds). Likewise, we should note that Judge Moss relied in part on expert testimony that used outputs from TRM’s data platform to assess and confirm the reliability of another analytic firm’s software, which was at issue in the case before him.
[3] “Machine learning (ML) is a branch of artificial intelligence (AI) and computer science that focuses on using data and algorithms to enable AI to imitate the way that humans learn, gradually improving its accuracy.” For a concise and accessible explanation of how machine learning works, see “Machine learning, explained,” MIT Sloan School of Management (April 21, 2021).
Sujit Raman is Chief Legal Officer at TRM Labs, a leading blockchain intelligence firm. Previously he was a partner at Sidley Austin LLP and the U.S. Associate Deputy Attorney General responsible for cyber investigations, crypto enforcement, and emerging technology issues. Thomas Armstrong is Head of TRM’s Compliance Advisory and former Head of Financial Crime Compliance Digital Assets at Goldman Sachs.
The views, opinions and positions expressed within all posts are those of the author(s) alone and do not represent those of the Program on Corporate Compliance and Enforcement (PCCE) or of the New York University School of Law. PCCE makes no representations as to the accuracy, completeness and validity or any statements made on this site and will not be liable any errors, omissions or representations. The copyright of this content belongs to the author(s) and any liability with regards to infringement of intellectual property rights remains with the author(s).