The assignment for this week is to play around DeepDream, a GAN network to transfer the style (or pattern) of an image, for example, this one:
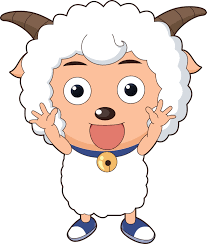
WORK
There are 5 parameters that are customizable in the step of generation in total. These are:
octave_n = 2
octave_scale = 1.4
iter_n = 15
strength = 688
layer = "mixed4a"
And today I am going to talk about my research and understanding of these parameters, as well as my tests and experiments.
octave_n
– Test Range: [1, 2, 3, 4, 5, 6]
– Test Outcome:

Form the test we can see the parameter determines the depth of deep dream. The larger octave_n
becomes, the deeper the render/transfer process will be. When it is set to 1, the picture is only slightly changed, the color of the sheep remains almost the same as its original source. However, when the parameter becomes larger, the contrast colors become heavier and the picture loses more features.
octave_scale
– Test Range: [0.5, 1, 1.5, 2, 2.5, 3]
– Test Outcome:

This parameter controls the scale of the deep dream. Although the contrast colors are not as heavier as the first parameter octave_n
, the size of each transfer point scales and affect a larger area. So, we can see from the last picture, the intersections of several transfers are highlighted.
iter_n
– Test Range: [10, 15, 20, 25, 30, 35]
– Test Outcome:

This parameter controls the number of iteration of the deep dream. In other words, it determines the times of image processing. When the number is smaller, the output woulld be more similar to its original input. When the number becomes larger, the output would be more ‘deepdreamed’.
strength
– Test Range: [300, 400, 500, 600, 700, 800]
– Test Outcome:

The strength
determines the scalar condition of each deep dream process. As we may see from the pictures above, the 6 transforms of the original picture are almost the same while only differ in the strength of colors (patterns). The higher strength outputs the sharper result.
layer
– Test Range: [“mixed3a”, “mixed3b”, “mixed4a”, “mixed4c”, “mixed5a”]
– Test Outcome:
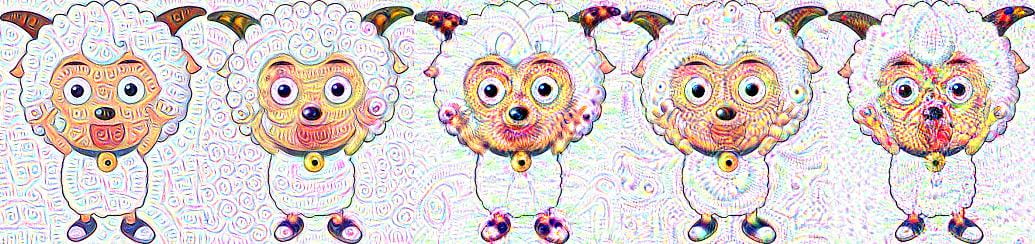
The layer
gives different patterns of the deep dream. It is also the pattern GAN used to train. So, each pattern would render different shape of DeepDream.