This week, I get to train cifar-10-CNN model for the first time. I trained it for 3 times, each time I modify a few parameters. The data I collected include acc, loss, val_acc, and val_loss for the training set, loss, acc, test loss and test accuracy for test set, time and batch for each training. I generated graphs with Tensorboard to demonstrate a clearer developing trend for my data.
The machine specs of my laptop are as follows:
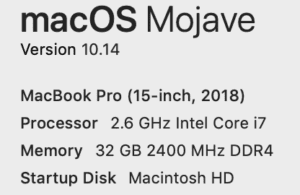
In my first time training, I adjust the number of epoch to be 5, hoping to receive the data and the result quicker, with the batch number of 2048, my laptop took 6m16s to finish training and testing. The acc value of the training set continually increases and the loss value continually decreases. The val_acc and val_loss are similar to the acc and the loss value. With the former continually increases and the latter continually decreases. In the testing set, it is using the model as it is last trained, with the loss and the acc values the same as the last pair of val_loss and val_acc values. Test loss and test accuracy are as follows, 0.3625 isn’t a high accuracy for my model.
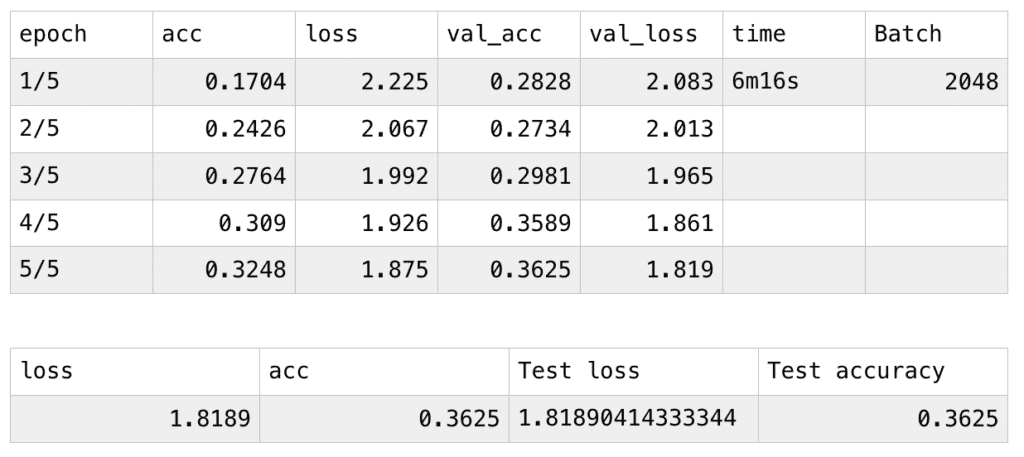
For the second time, I changed the number of the epoch from 5 to 10. The batch number remains 2048. Accordingly, the total time for training and testing increases to 14m48s. This time, the acc and the loss value for my training set follow the previous pattern when the number of epoch is 5, however, there are two glitches in the changing val_acc and one in val_loss, for the former value, it should be in the pattern of continuously growing, however, the data decreases when the 8th and the 10th epoch is finished; for the val_loss values, it should be decreasing continuously, however, the value bounced back a little when the 8th epoch is finished. With the model after the last training, I received a lower test loss and a higher test accuracy which is 0.4114, indicating that the performance didn’t improve too much.
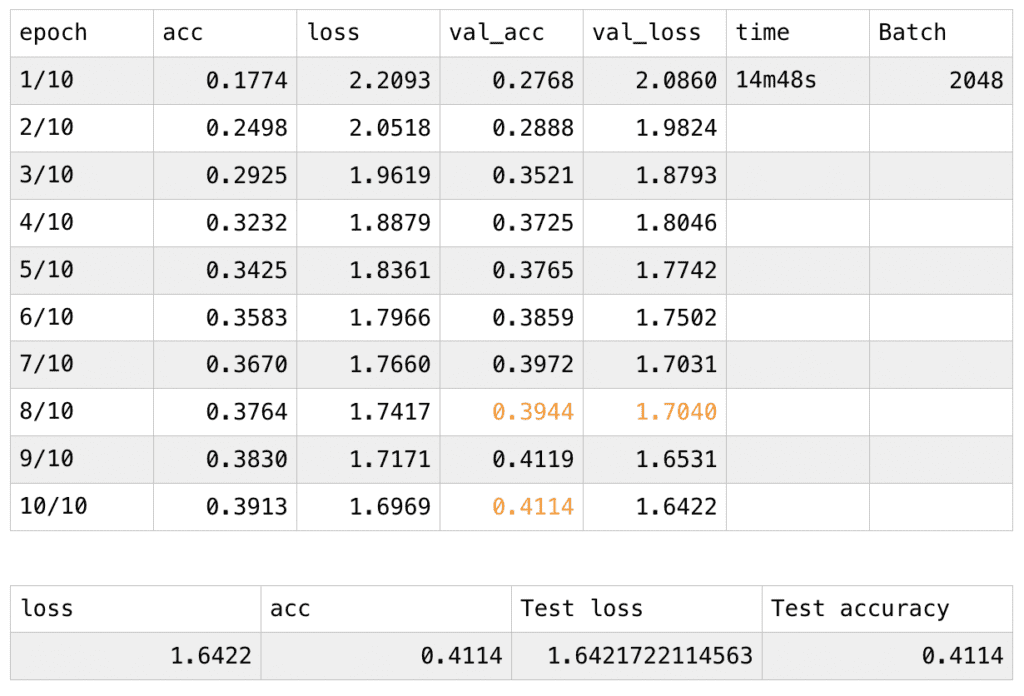
For my third time training, I kept the number of the epoch at 10 and decreases the batch value from 2048 to 32. This time the total time for my training and testing lasted for 17m12s. There’s one glitch for val_acc and val_loss: when the 8th epoch is finished, the values of val_acc and val_loss didn’t follow the previous pattern. Nevertheless, after applying the final version of the trained model to the testing set, I received the best pair of test loss and test accuracy figures of my three pieces of training: the test accuracy increases a lot compared to the second result.
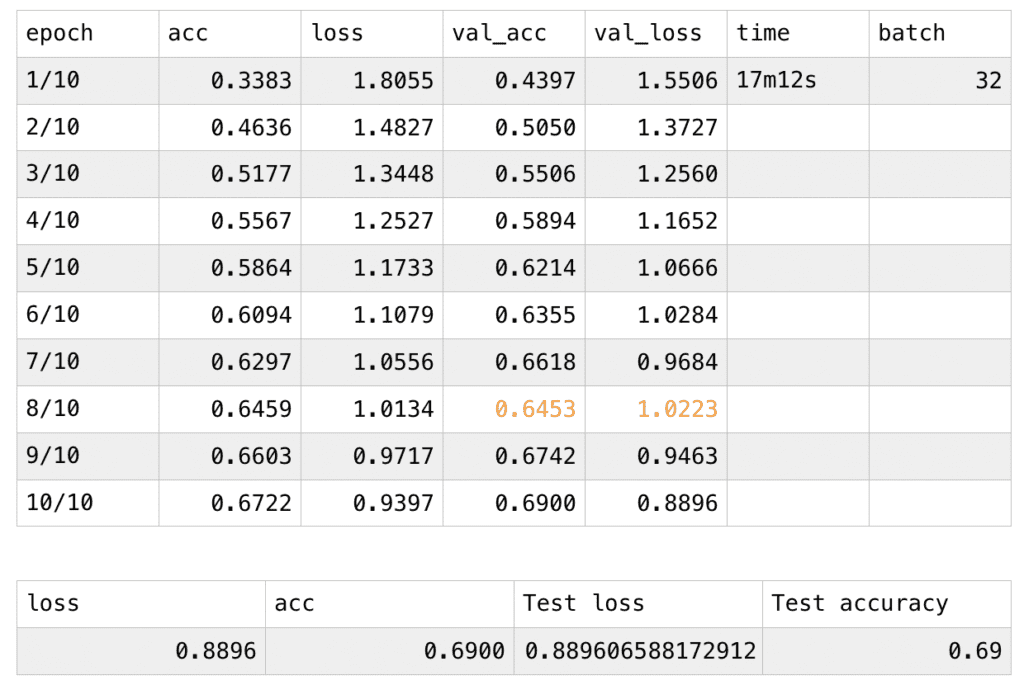
Concluded through Tensorboard:
The following is the graph generated on Tensorboard, the shortest thread indicates the first training, the blue one is the second and the red one is the third. It can be concluded that when the number of epoch changes, the values remain similar (see the shortest thread and the blue thread). Whereas when I make changes on batch numbers, the data vary vastly and the results I receive distinguish in a large amount (see the blue and the pink threads). It may result from the fact that the change I made between the first and the second training on the number of epochs doesn’t vary much (from 5 to 10).
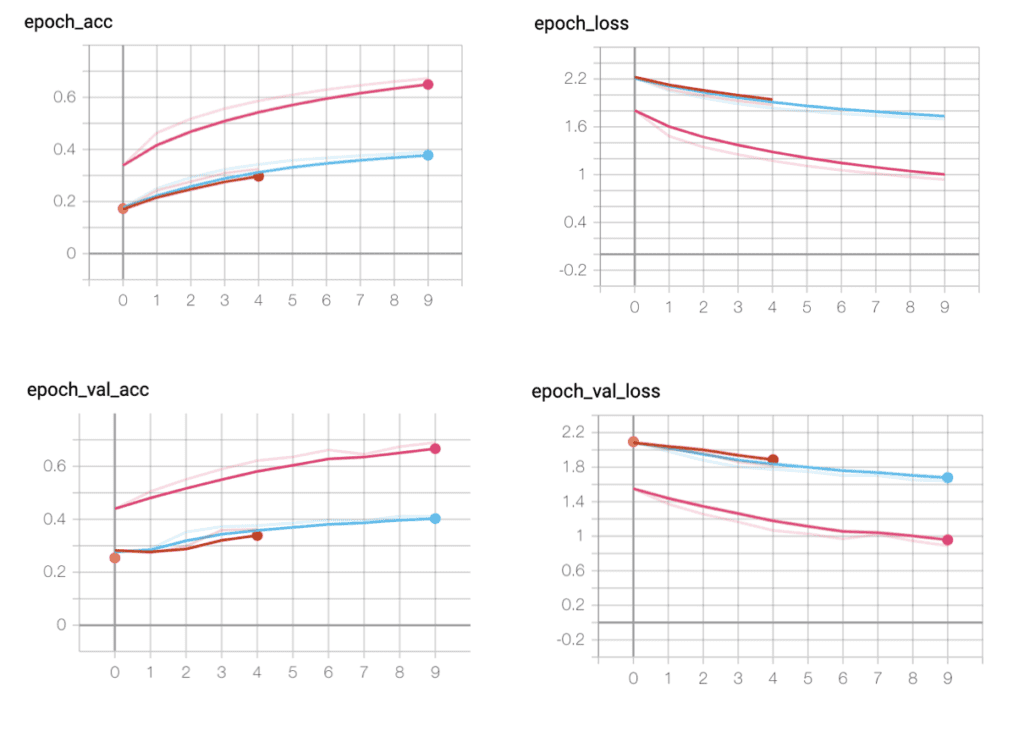
My explanations:
The results can’t be concluded as the higher the number of epochs is, the more accurate this model can be, or, the smaller a batch is, the more accurate the model is. But theoretically, one epoch can be interpreted as one thorough go-over of all training resources, the model refines itself a bit when every epoch is finished, and the model should be at its best state when val_acc stops changing. With the changing in the numbers of a batch, the smaller the batch is, the more iterations it needs to go through, and the training the model has to go through.