Introduction
For this week’s assignment, we are training a CIFAR-10 CNN. Before doing anything involving actual training, I first wanted to understand the CIFAR-10 dataset because I’ve never worked with it before. I read that the dataset contains 60,000 images in total (the training set is only 50,000), with each image at 32×32. These are broken down into 10 classes: airplanes, cars, birds, cats, deer, dogs, frogs, horses, ships, and trucks. Each class contains 6,000 images.
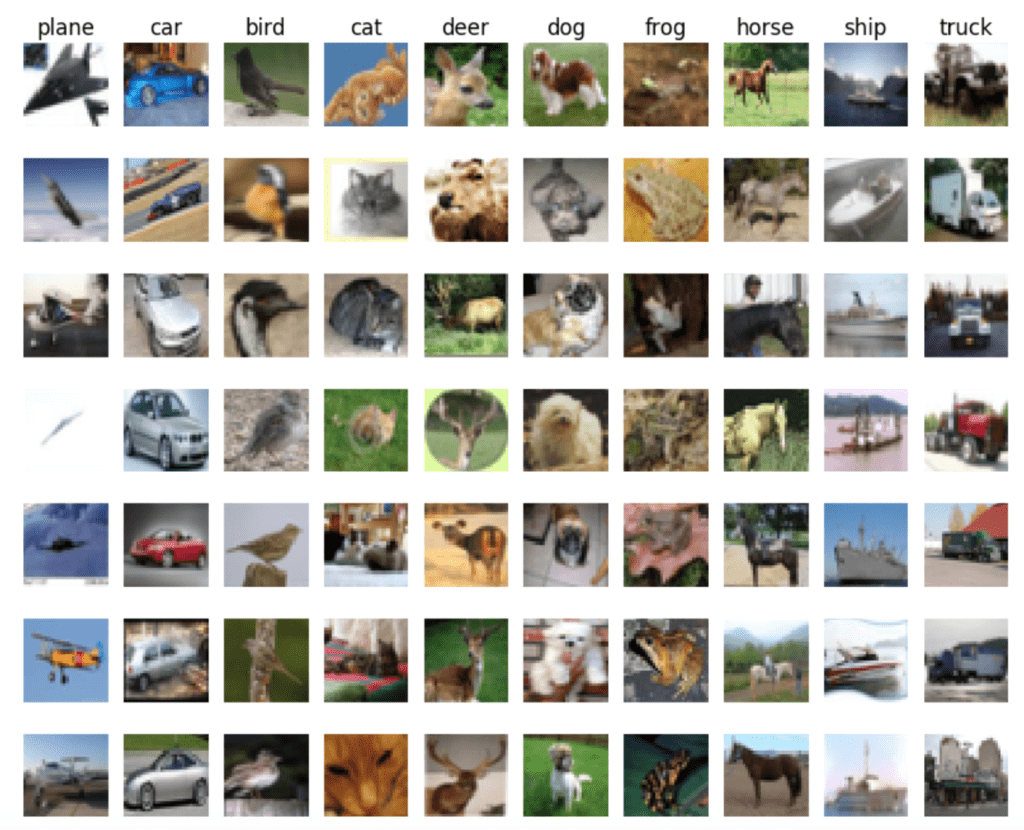
Machine Specs
Tests
I ran 3 different tests to test the relationship between epochs and batch size, and its effect on accuracy. Test 1 is high epoch, high batch. Test 2 is low epoch, high batch. Test 3 is low epoch, low batch.
1: (Epochs: 100, batch size: 2048, 20 hours)
The first test I did (not sensibly) was to just run the program. It was really painful but I wanted to see it through to see what it could do. I knew this was going to take forever to complete, so I tried running it when I was asleep. I forgot that my computer also goes to sleep very quickly when I’m not using it. I had to come back to this a lot to wake my computer up, so as a result, this took me 20 hours. This resulted in a 0.6326 accuracy. I was pretty surprised at this, considering the testing we did in class resulted in much higher accuracy, and much faster.
2: (epochs: 10, batch size: 2048, 25 minutes)
Thinking that it was the high number of epochs that took so long to process (even if it would have been continuous), I assumed that lowering them to 10 would go very fast. It definitely cut down on the total amount of time, but it seemed to be processing at about the same rate as the first test. The final result is a 0.4093 accuracy.
3: (Epochs: 10, Batch size: 256, 20 minutes)
Finally, I decreased the batch size as well, meaning testing low epoch, low batch. This had surprised me that despite testing a much lower batch size than in test 2, it took about the same amount of time. Even more surprising was the fact that it resulted in a 0.5453 accuracy, meaning that the lower batch size produced a more accurate result.
Conclusion
I’m still a bit confused about the different aspects that go into CNN training, and especially how their relationships with each other affect the outcomes. But at the very least I learned the amount of time it would take to try to train the CIFAR-10 dataset. I’m still not totally sure why it took so much more time for a less accurate result than in class, especially since the Fashion-MNIST dataset has an almost equal number of images as CIFAR-10. On quick evaluation, I see that each image in Fashion-MNIST is only 28×28, whereas CIFAR-10 is 32×32. I wonder if that has something to do with it, but that difference still seems pretty small for such a big gap in the results.