Title
The Default
Project Description
The video project raises questions related to gender bias in AI/ML not from a human’s perspective, but from the vantage point of a machine learning model being trained. Inspired by In visible Women: Data Bias in a World Designed for Men by Caroline Criado Pérez, the video looks at how existing inequalities are being perpetuated through training data to from an “AI” that is a mere reflection of the biased world it emanated from.
Perspective and Context
To start with why I particularly want to dig into this topic, this is where my personal interest lies in. I consider gender as something related to each one of us yet so easily produces bias, prejudice, stereotypes, physical and mental harms. I’ve done related works like an interactive website about gender stereotypes and fluidity, 3D printed objects exploring female health, and held relative events as well.
After taking Machine Learning for Artists and Designers, I surprisedly learned that the future technological development, if not regulated and developed with caution, can only worsen how people view gender. Even with our ideas becoming more progressive and inclusive, we are inevitably influenced by the advertisements tailored to us by algorithm, the auto decision making of AI (e.g. whether to reject an applicant’s resume), different medical treatments, based on the gender that ML perceives us as, not to mention the training data it receives is already skewed (you may be perceived very wrongly).
We have also discussed about bias-related issues in class, which can be great starting points for me to start the project. For instance, in the documentary Coded Bias that we watched at the beginning of the semester, MIT Media Lab researcher Joy Buolamwini discovers that facial recognition does not see dark-skinned faces accurately, she then embarks on a journey to push for the first-ever U.S. legislation against bias in algorithms. While we were learning about Stable Diffusion and other AI generative tools, we could see that AI has its own understanding of certain professions like 3D printing expert and tool review YouTuber, and when a girl asked AI to polish her Linkedin profile photo, it made her more Caucasian by changing her eye and hair color.
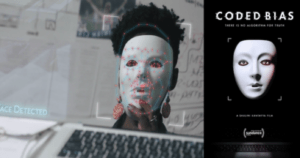
Design Process
I first started with a video of Caroline Criado Pérez who wrote the book Invisible Women, in which she gives real-life cases where the gender data gap has seriously affected women’s lives. For example, the fact that in most drug tests, male animals are used with excuses that “the menstrual circles will affect the findings”, which leads to the inaccuracy in the prescription of women. There are heart arrhythmia drugs that are more likely to trigger a heart attack in the first half of a woman’s menstrual circle. In the end, she points out that the medical, hiring, and public transportation data in real like are helplessly biased towards one gender, not to mention having an algorithm that receives data and gets better and better at being biased.
In the article Examining How Gender Bias is Build into AI, according to Manasi and Dr. Panchanadeswaran, using a data set that lacks diversity and information on certain demographic categories like women of color, might skew results. They also say that research has repeatedly shown that AI models are often trained on male-centric data, which in turn yields results that misidentify women and people of color.
Done reading more scholarly articles related to the topic, I summed up a few cases that indicate the negative outcomes of using biased machine learning:
- Google translate: entering a gender-neutral sentence in Hindi such as “Vah ek doktar hai” (“That is a doctor”) gets translated in English as “He is a doctor.” Similarly, “Vah ek nurse hai”(“That is a nurse”) gets translated in English as “She is a nurse.” While, it is true that there are more male doctors than female doctors due to rates of burnout (Poorman2018).
The algorithm is interpreting it as a stronger association of males with doctors and females with nurses. Thus, the stereotype that doctors are male and nurses are female is perpetuated. job search algorithms as well. Incorrectly learning a social disparity that women are overrepresented in lower paying jobs as women’s preference for lower paying jobs, the algorithms recommend lower paying jobs to women than to men (Bolukbasi et al. 2016).
- If a firm uses reviews containing gender bias to design products and promotions, recommend products to consumers, determine how to portray individuals in a commercial, segment consumers etc. then it could lead to biased recommendations, messages or insensitive portrayals. Specifically, recommendation using biased reviews would result in learning and using consumer vulnerability against female consumers. Moreover, if these reviews are used to provide recommendations in the marketplace, it would result in women being recommended less career-oriented products e.g. less online courses, job advertisements, or even less paying jobs (e.g., Bolukbasi et al. 2016).
- Amazon decided to use an AI-powered recruiting tool in 2015. The automated tool was trained to identify promising applicants by observing patterns in resumes submitted to Amazon over a 10-year period. It then would assign job candidates scores ranging from one to five stars. However, most of the resumes came from men given that the tech industry is notoriously male-dominated.
Based on the data it used, the system taught itself to prefer male candidates over female ones, and penalized resumes that included words like “women’s”, such as “women’s chess club captain”. It also downgraded graduates of two unnamed all-women’s colleges and gave preference to what Reuters referred to as “masculine language” and the use of stronger verbs such as “executed” or “captured.”
……
Based on the findings, most of the critical voices focus on how the data gets into machine learning is skewed towards males in the first place, and the algorithms keep learning data that humans feed them. There are voices advocating unbiasing the ml system, such as augmenting the training data by replacing the gendered pronouns and words with the opposite gender and blanking out names, so that the training data becomes gender neutral and doesn’t relate gender characteristics with certain names. However, it hasn’t come to the majority of people’s mind, especially those who design and develop the system.
Since there are already plenty of videos explaining gender bias in machine learning, I decided to do something different, more like telling a story from the perspective of a machine learning model. It is innocent, trying to defend itself from the outside criticism yet feeling confused and down. The narrative should be calm, while the audience can feel the indifference and helplessness in the tone depending on how they perceive the video.
For the visual, I wish to find a balance between informative and aesthetic. I don’t want it to become an educational short video, rather I hope the audience can feel the emotion hidden in lines. A head model was used throughout the shooting to indicate the machine learning model, with black and white movie clips in the background. Besides the information I concluded above, I also collected plenty of online images and generated a few using Stable Diffusion, an ai generative tool.
I name the project The Default to indicate that machine learning has learnt that male is the default gender of human beings based on the millions of information it received and it chooses to lean towards the default image that it assumes in each and every decision making.
Technical Development
The shooting
The tone is set to be grey plus a bit of black and white, thus I chose a white setting and a white head model, with a cold-tone lighting. Through panning and tilting the camera, I filmed the model from different angles, giving close-ups to its side and face.
At the beginning and end (00:20 and 02:23), there are flashlights on the model. I used the light switch to achieve the effect, indicating the projection of the human world on machine learning.
Most of the clips were done on a tripod, yet I intensionally took the camera off the tripod and handheld it for a few clips to create a bit of shaking. Through the shaking the audience could feel the emotion changes of ml.
The editing
I used multiply in the video editing software to show some of the critical newspaper (00:25) of bias in ml, which is like a crime imprinted on its body. Techniques permitting, i would not just use multiply but use uv in 3d modeling software to stamp the newspaper on the model.
Some of the images are flashy as I wanted to give a quick impression instead of having the audience clearly read the information on them, so that they could be more focused on what the ml model is saying.
Website screenshots usually overlay old movie clips to indicate a contrast between the biased human society and the innocent algorithm. The biased messages today are more implicit, while I wanted to display them in a blunt way to confront what’s deep down in people’s minds.
Suggestions & Improvements
In the first user-test, Phoebe suggested that fewer informative images should be shown because it sort of damages the aesthetic of the video and makes it a slideshow instead of a video essay. Plus my friend Luna thought that it was hard for people who first saw the images to understand their meanings in a short while. Thus I replaced them with more intuitive pictures like the female and male icons (00:55) and the model shots (01:43).
Gottfried and Leon suggested that there were disconnections between the subtitle and voice-over, and sometimes the video required the audience to both read and listen at the same time, which could be challenging. I rearranged the script and increased the time length that each piece of information stays (1:51). As an editor quite familiar with the video, sometimes it is difficult for myself to realize there is too much going on in a short time scope, and having fresh eyes indeed helped me discover quite a few problems that I ignored.
Taojie, another friend of mine, raised an interesting question done watching the video. She said that though I was trying to portray a gender-neutral model the whole time, this model still looked like a female to her because of the angle that the head rotated and the curves in its shoulders (00:22). I could use ai animation tool to generate other parts of the model to indicate that it is gender neutral or it is actually a male. A similar aspect was raised by Luna as well. She believed that the logic of machine learning strengthened the stereotypes of different genders, and someone perceived as a male by ml (with distinguish male features) may happen to be someone identify as a female, while it is hard to be studied by ml and harms gender diversity. This is a perspective that I long to touch upon while didn’t display and explain very well in the video. If given a future chance, I would focus on the hidden features (psychologically, socially) of humans that are difficult for ml to learn about so that it just keeps reinforcing the old, visible features and does harm to the gender diversity development in the future.
I also went to professor Wuwei Chen for advice. He asked whether the neck of the model is intensionally made longer and longer as the video proceeds. While I actually didn’t notice it when editing. Wuwei suggested that if one or some parts of the model is gradually distorted as it is fed more and more information from the real world, it could be a good metaphor indicating what’s inside the model is distorted, which can be done through generative ai animation. In the end, he suggested that I could leave an open ending, where the audience take a guess of what’s waiting in the future for humans and machine learning. Using Runway, I inserted seconds of ai animation in the end (02:26). The model seems to be moving its head and saying things that we don’t know, which is also like there is a real human being, or some existence that acquire real emotion, trapped inside a model that seems neutral and indifferent. I additionally overlaid several voices on top of the original voice-over, indicating the crowds of machine learning models being influenced.
Conclusion
In general, I very much enjoyed the process of doing research and producing a video related to both the course material and what I am passionate about. I learned a lot and received genuine feedbacks from my peers and professors. I wouldn’t say the video project is well polished as I believe it only touches upon a narrow aspect of the issue and there is definitely my personal bias in it even I tried to avoid as much as possible. I would very much like to turn the project into something more intuitive and interactive through a website or other medium if I have time.
Reference
https://www.youtube.com/watch?v=qpYyI9Tdtc4
https://www.youtube.com/watch?v=hB92hQeCEAw
https://www.youtube.com/watch?v=Z7-DjqgO2ws
https://www.adelphi.edu/news/examining-how-gender-bias-is-built-into-ai/
https://www.frontiersin.org/articles/10.3389/frai.2022.976838/full
https://www.lexology.com/library/detail.aspx?g=c384fd7d-0e20-4724-b5e4-a7927d016f49
https://hbr.org/2019/05/voice-recognition-still-has-significant-race-and-gender-biases
https://cratesandribbons.com/2013/01/27/why-the-default-male-is-not-just-annoying-but-also-harmful/