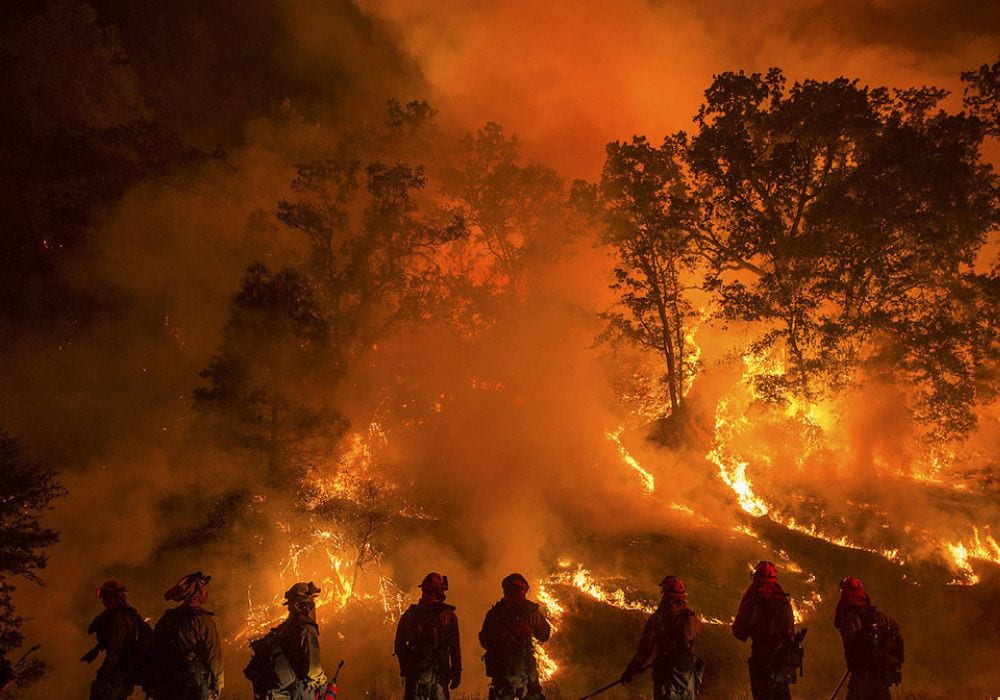
Increased incidences of natural disasters such as wildfires are an expected byproduct of climate change. Wetter winters, drier summers, and hotter temperatures are predicted for North America’s west coast in coming years, with recent atmospheric rivers putting California in even further danger of a worsened wildfire season. In preparation for a major fire disaster, California must leverage modern technologies such as wildfire spread models.
High-intensity fires as seen in recent years have innumerable consequences beyond environmental destruction. In addition to potential burns and injuries, wildfires severely impact pulmonary function and strain cardiac conditions. Wildfire smoke can also endanger pregnancies and lead to pre-term birth and low birth weight. Aerosols due to biomass burning can even affect atmospheric chemistry, with a recent study showing chemical processes in the atmosphere involving wildfire smoke contribute to mid-latitude ozone depletion.
Smoke can be transported on continental scales to areas far removed from wildfire activity, further magnifying harmful effects. The long-range transport of wildfire smoke points to the critical coupling of wildfires with the atmosphere, where both the spread of the fire and the state of the atmosphere have a strong influence on one another. Large wildfires can also lead to atmospheric phenomena such as firestorms, where heat from a wildfire can create its own wind system.
The importance of forecasting wildfire behavior has led to the creation of a wide array of computational models for predicting the course of a fire. These wildfire models range from simple models for fire spread to complex models which consider coupling to the atmosphere. For these models to be useful, they must be both accurate and efficient enough to use in real-time, without relying on large computational resources.
One popular wildfire spread model is the coupled atmosphere-wildfire model WRF-SFIRE. It combines the Weather Research and Forecasting Model (WRF) with SFIRE, a fire spread model based on the semi-empirical fire spread rate formula introduced by Richard C. Rothermel in 1972. The fire spread model SFIRE accounts for terrain, fuel parameters, and environmental conditions, allowing it to predict wildfire spread for a range of conditions. WRF-SFIRE can further be coupled to the atmospheric chemistry model WRF-Chem, which allows for more complex simulations of smoke dispersion and atmospheric chemistry, critical for air quality considerations.
The coupled atmosphere-wildfire model WRF-SFIRE uses surface winds from the atmospheric model, along with terrain and fuel information, to drive fire spread. The model then captures the output of heat from the fire to the atmosphere. The heat released by the fire influences the state of the atmosphere, which then feeds back into the fire spread model through the altered surface winds. Smoke adds yet another variable to be considered.
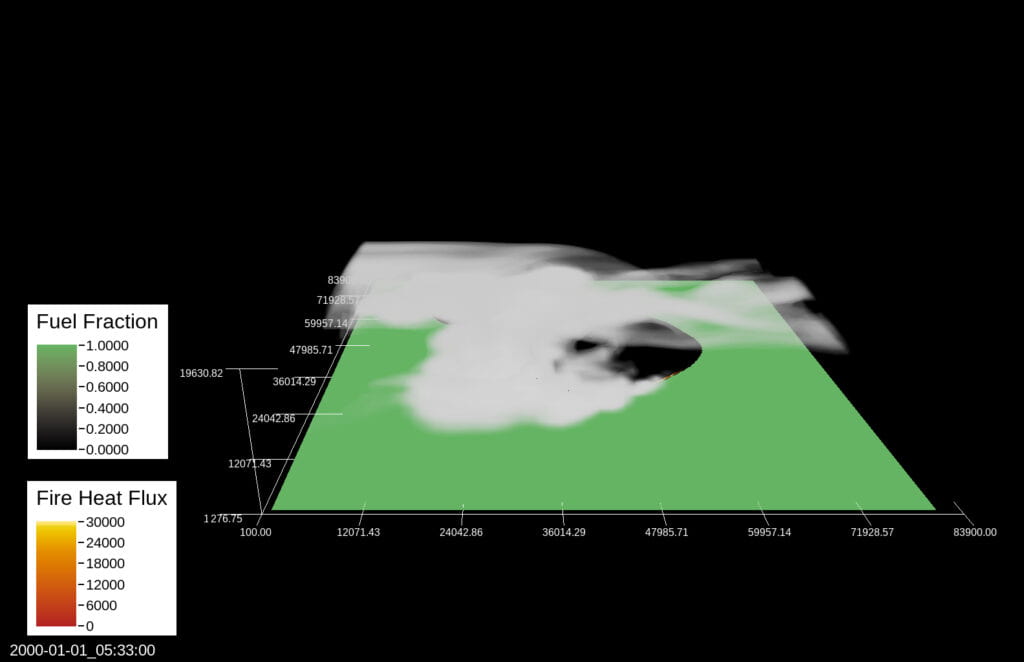
Over the last decade, WRF-SFIRE has been refined with the addition of features expanding its applicability to real-world cases, including advances such as the ability to initialize simulations from a perimeter rather than a single ignition point. Remote sensing of wildfires in the form of satellite measurements has also improved immensely in both quality and availability. Satellite detections of active fire locations are collected approximately four times a day by the Visible Infrared Imaging Radiometer Suite (VIIRS) aboard the Suomi-NPP and NOAA-20 weather satellites, with additional detections coming from its predecessor MODIS and geostationary satellites from the GOES program.
With the availability of satellite measurements of wildfires, there is significant interest in incorporating these measurements into wildfire models. One recent application uses a machine learning method, known as a support vector machine (SVM), along with satellite detections to predict the times a wildfire front arrived at any given location over the course of a fire, known as the fire arrival times. These fire arrival times contain information about the history of wildfire spread for a given fire. By providing this information to WRF-SFIRE, the model can initiate forecasts from the current state of a wildfire and produce forecasts of future wildfire spread, consistent with measurements.
While the SVM method provides a way to infer fire arrival times from satellite measurements, it does not explicitly account for the physical processes inherent in the fire-spread process, or the randomness associated with wildfire systems. Earlier this year, University of Southern California (USC) Mechanical Engineering Ph.D. student Bryan Shaddy presented work on “Deep-Learning Based Estimation of the Initial Atmospheric State for Wildfire Prediction” at the American Meteorological Society’s 103rd Annual Meeting, completed alongside colleagues at USC, San Jose State University, University of Colorado Denver, University of Utah, and the Colorado State University Cooperative Institute for Research in the Atmosphere.
This work aims to improve upon the SVM method for determining fire arrival times from satellite measurements by accounting for the probabilistic relationship between measurements and true fire states, in addition to incorporating some physics into the predictions via the training data used. This method utilizes ideas from probabilistic deep learning, in particular conditional Wasserstein Generative Adversarial Networks (cWGAN). The aim is to improve the accuracy of predictions of fire arrival times, in addition to providing insights into prediction uncertainty.
As work continues to improve wildfire modeling capabilities, the ability to produce robust and reliable emergency plans should only improve. Currently, Los Angeles County is ranked “very high” in wildfire risk, expected annual loss (EAL), and social vulnerability by the Federal Emergency Management Agency (FEMA) National Risk Index while community resilience (which rates preparation, adaptability, and recovery from natural hazards) ranks “very low.” Additionally, the Los Angeles Emergency Operations Plan’s Brush Fire Hazard Specific Annex has not been updated since March 2018.
In this semester’s NYU Global Health Disaster Preparedness & Response cohort, Joanna Horvath, Victoria Sevilla, Hyacinth Burrowes, and Gangao Chen are working on a Wildfire Outbreak Emergency Operations Plan for Los Angeles County.
Article by Bryan Shaddy and Victoria Sevilla. View the full list of references here.
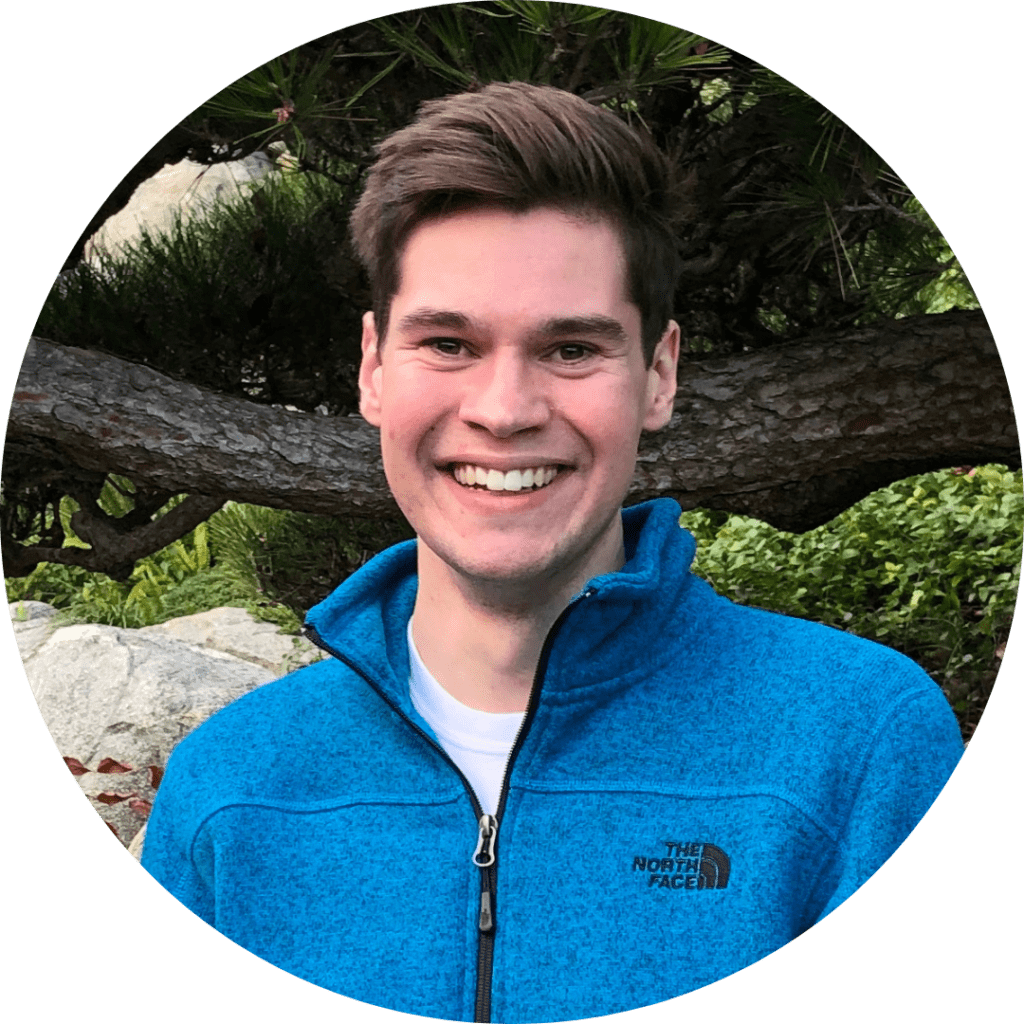
Bryan Shaddy is a second-year mechanical engineering PhD student at the University of Southern California (USC). Currently, he is working on applying machine learning-based methods to improve wildfire modeling capabilities. Prior to joining USC, he graduated from the University of California, Riverside (UCR) in 2021 with a BS in Mechanical Engineering. At UCR, he worked on modeling the motion and dispersion of sea ice in the Arctic Ocean due to stochastic environmental forces.
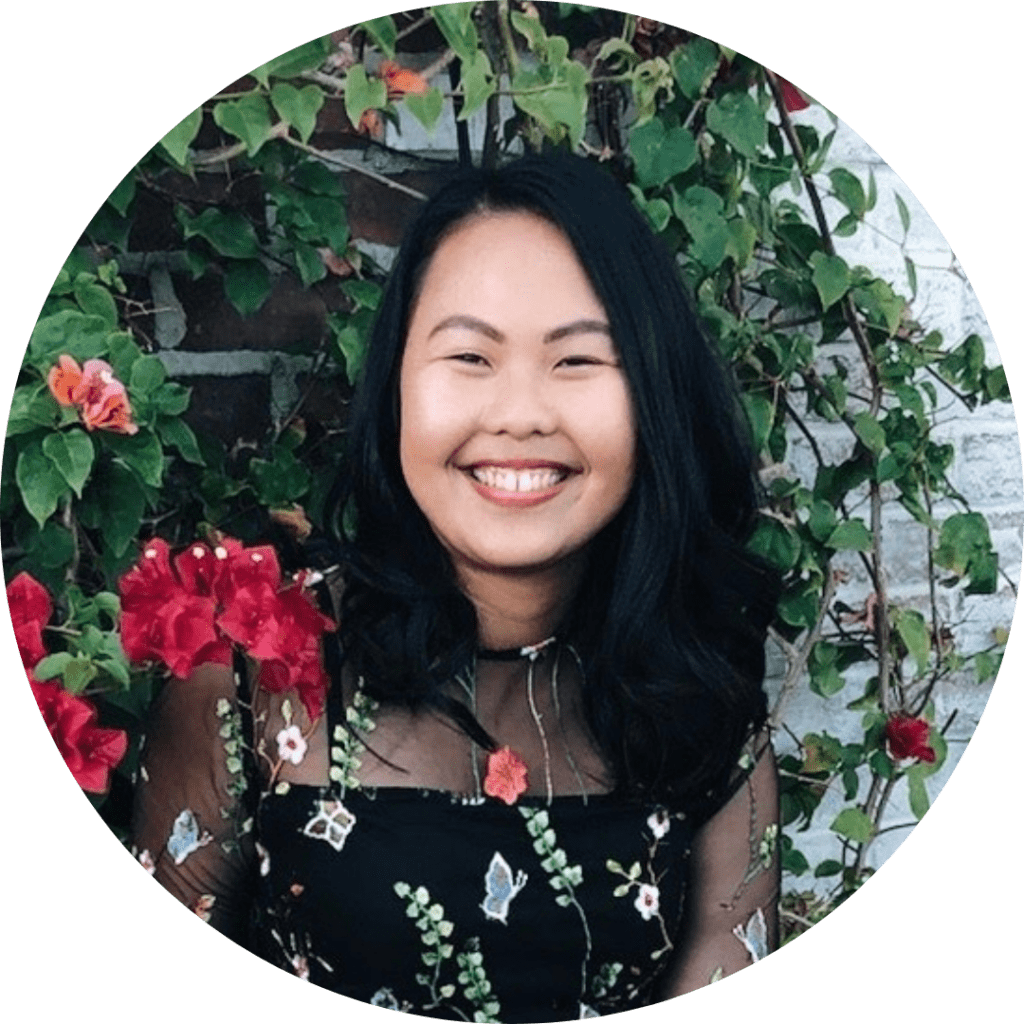
Victoria Sevilla is a first-year Master of Public Health student at NYU School of Global Public Health. She received her Bachelor’s degrees in Global Health and Biological Sciences at the University of Southern California (USC). Victoria works part-time at USC Viterbi School of Engineering’s Department of Aerospace and Mechanical Engineering (AME) where she is the Co-Chair of the AME Publicity Committee and a member of the AME Diversity, Equity, & Inclusion (DEI) Committee.