One of our recent works entitled “LARRI: Learning-based Adaptive Range Routing for Highly Dynamic Traffic in WANs” was accepted by the IEEE International Conference on Computer Communications (INFOCOM 2023). Congratulations!
In this work, we developed a learning-based adaptive range routing scheme to accommodate dynamic future traffic fluctuation in the networks. The key insight is to directly predict a routing for future traffic scenarios by combining Supervised Learning (SL) and Graph Neural Network (GNN) techniques. This work was presented at the INFOCOM conference in Hoboken, NJ, USA, on 5/17/2023.
Project Overview:
(1) Existing problem: Traditional TE cannot adapt to unexpected traffic fluctuations in the future(2) LARRI: Directly predict appropriate range routing strategies based on historical traffic demands to accommodate future traffic variations
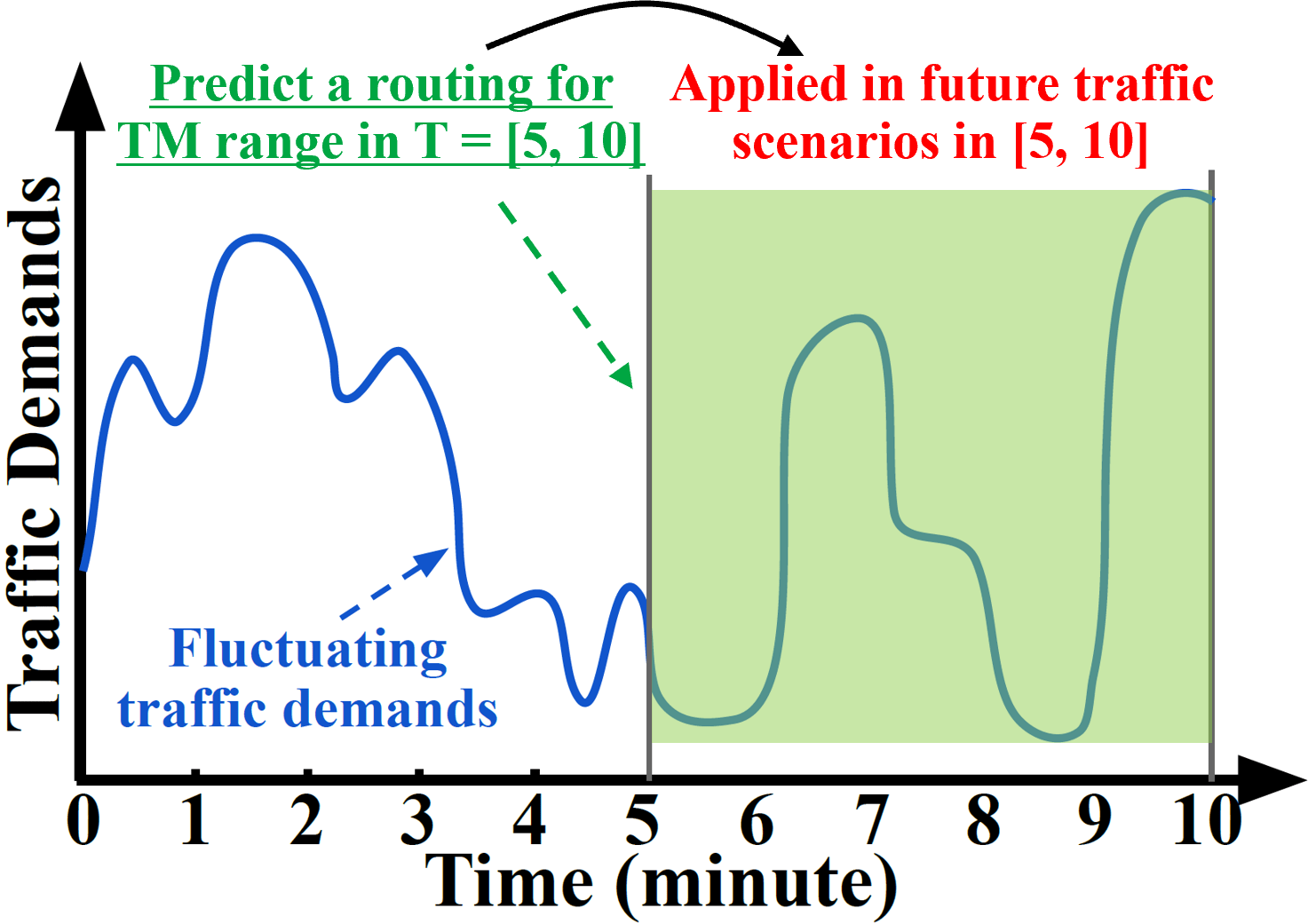
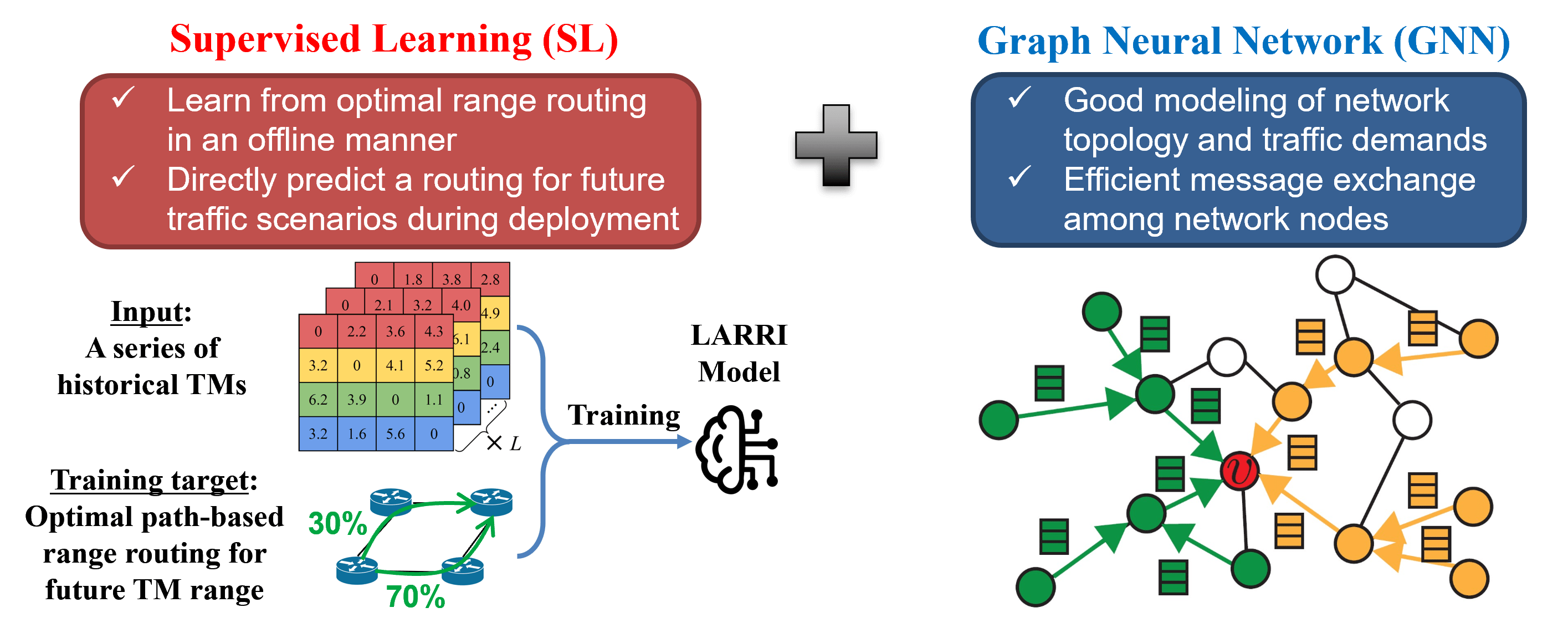
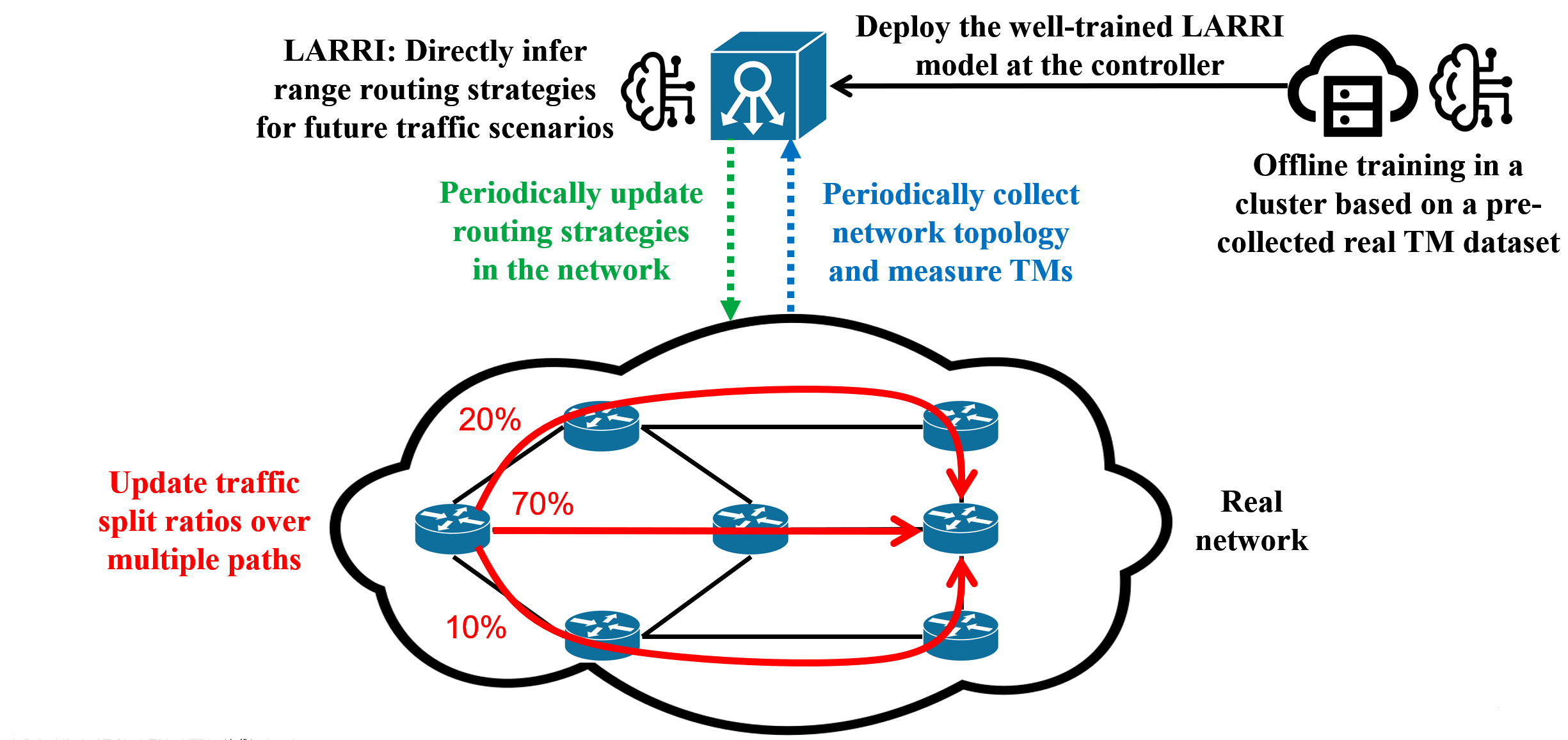
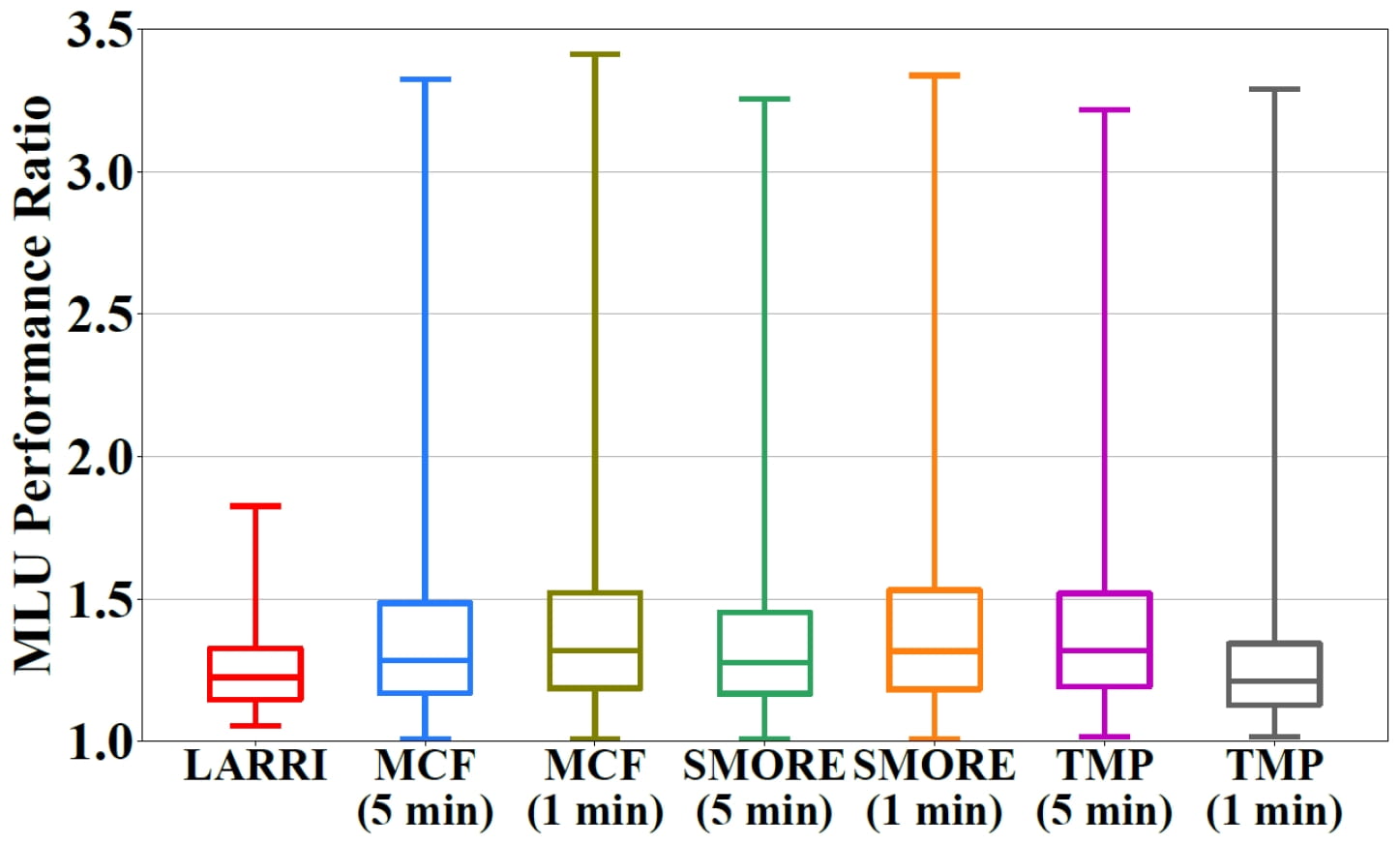
Abstract:
Traffic Engineering (TE) has been widely used by network operators to improve network performance and provide better service quality to users. One major challenge for TE is how to generate good routing strategies adaptive to highly dynamic future traffic scenarios. Unfortunately, existing works could either experience severe performance degradation under unexpected traffic fluctuations or sacrifice performance optimality for guaranteeing the worst-case performance when traffic is relatively stable.
In this paper, we propose LARRI, a learning-based TE to predict adaptive routing strategies for future unknown traffic scenarios. By learning and predicting a routing to handle an appropriate range of future possible traffic matrices, LARRI can effectively realize a trade-off between performance optimality and worst-case performance guarantee. This is done by integrating the prediction of future demand range and the imitation of optimal range routing into one step. Moreover, LARRI employs a scalable graph neural network architecture to greatly facilitate training and inference.
Extensive simulation results on six real-world network topologies and traffic traces show that LARRI achieves near-optimal load balancing performance in future traffic scenarios with up to 43.3% worst-case performance improvement over state-of-the-art baselines, and also provides the lowest end-to-end delay under dynamic traffic fluctuations.
Publication:
- [INFOCOM ’23] Minghao Ye, Junjie Zhang, Zehua Guo, and H. Jonathan Chao, “LARRI: Learning-based Adaptive Range Routing for Highly Dynamic Traffic in WANs,” IEEE International Conference on Computer Communications (INFOCOM), 2023. (Selected as one of the five fast-tracked papers for submission to IEEE/ACM Transactions on Networking. Acceptance rate: 19.2%, 252/1312) [Paper URL] [PDF]