Traffic Engineering (TE) may render high time complexity when generating and updating a large number of forwarding entries in the network. To mitigate such routing update overhead, we developed a 2-stage Reinforcement Learning (RL)-based TE solution to identify a flexible number of critical destination-based forwarding entries to be updated in different traffic scenarios. As a result, we only need to compute the optimal traffic split ratios for these critical entries and update them accordingly to achieve close-to-optimal performance, while reducing the entry updates by up to 99.3% on average.
Project Overview:
(1) Our idea: Mitigating routing update overhead by only updating some critical entries at some critical nodes to reroute traffic and improve network performance
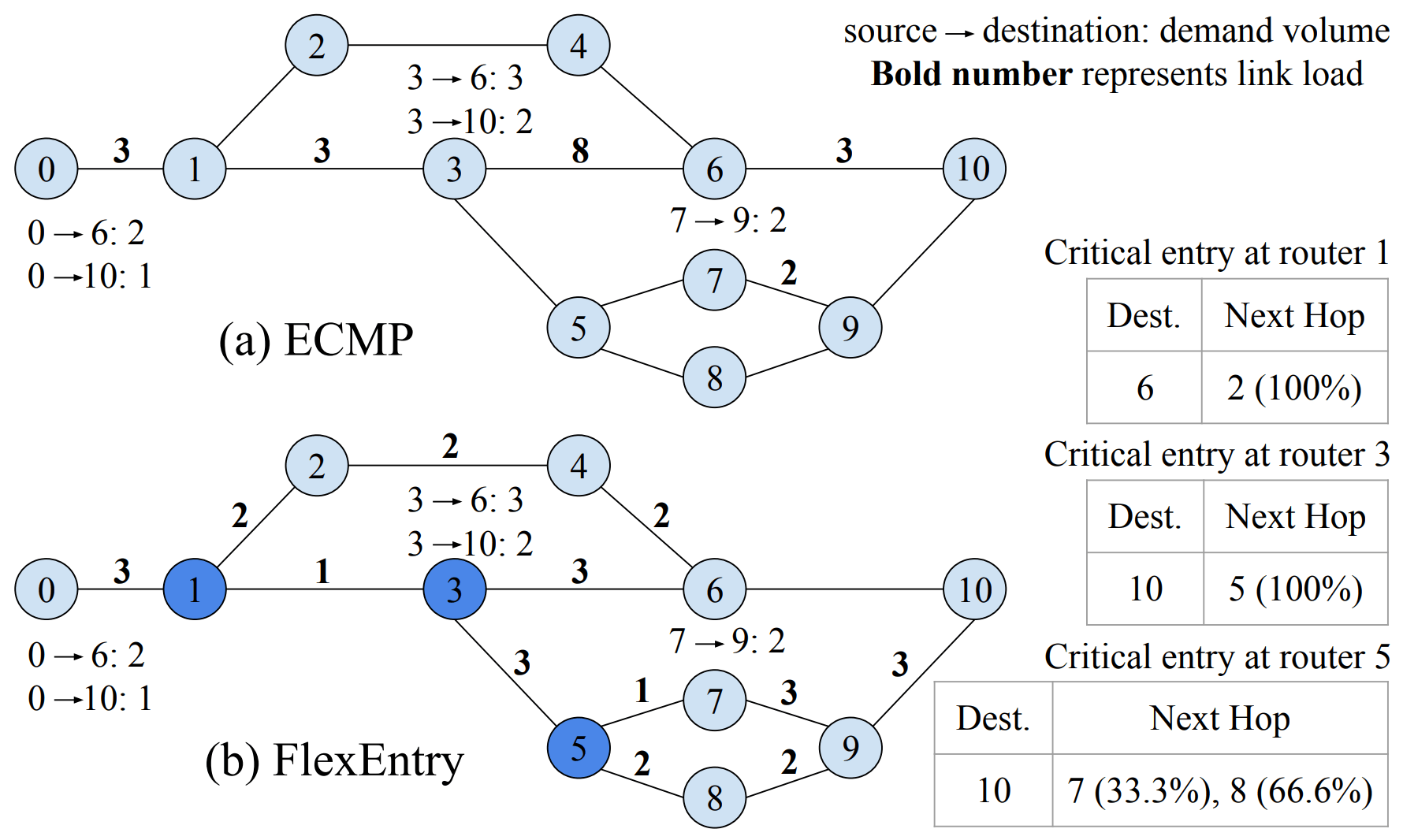
(2) FlexEntry: Reinforcement Learning (RL) + Linear Programming (LP) combined approach
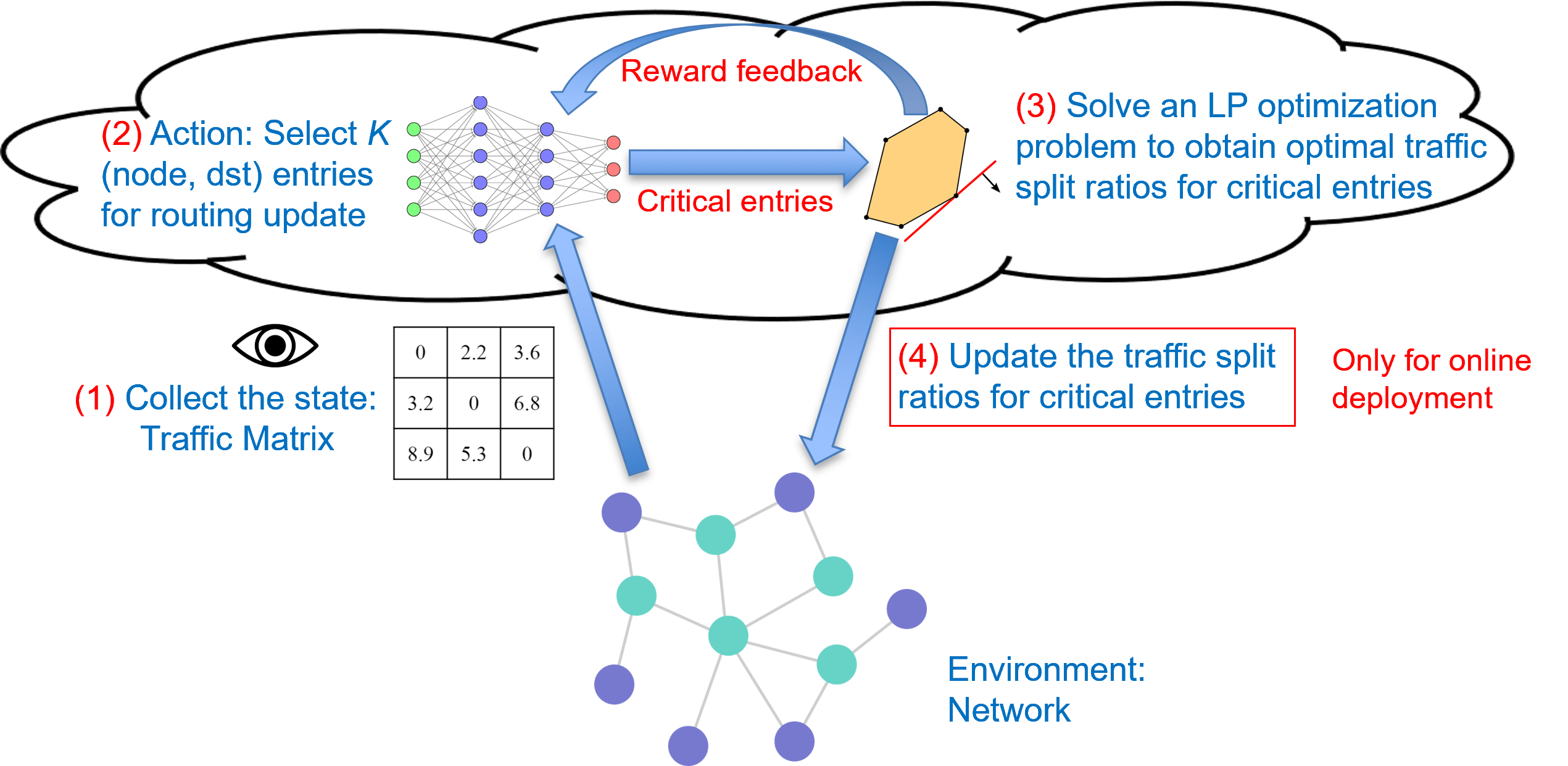
(3) Stage 1: Train multiple RL sub-models with different numbers of critical entries 𝐾 to be identified in different traffic scenarios
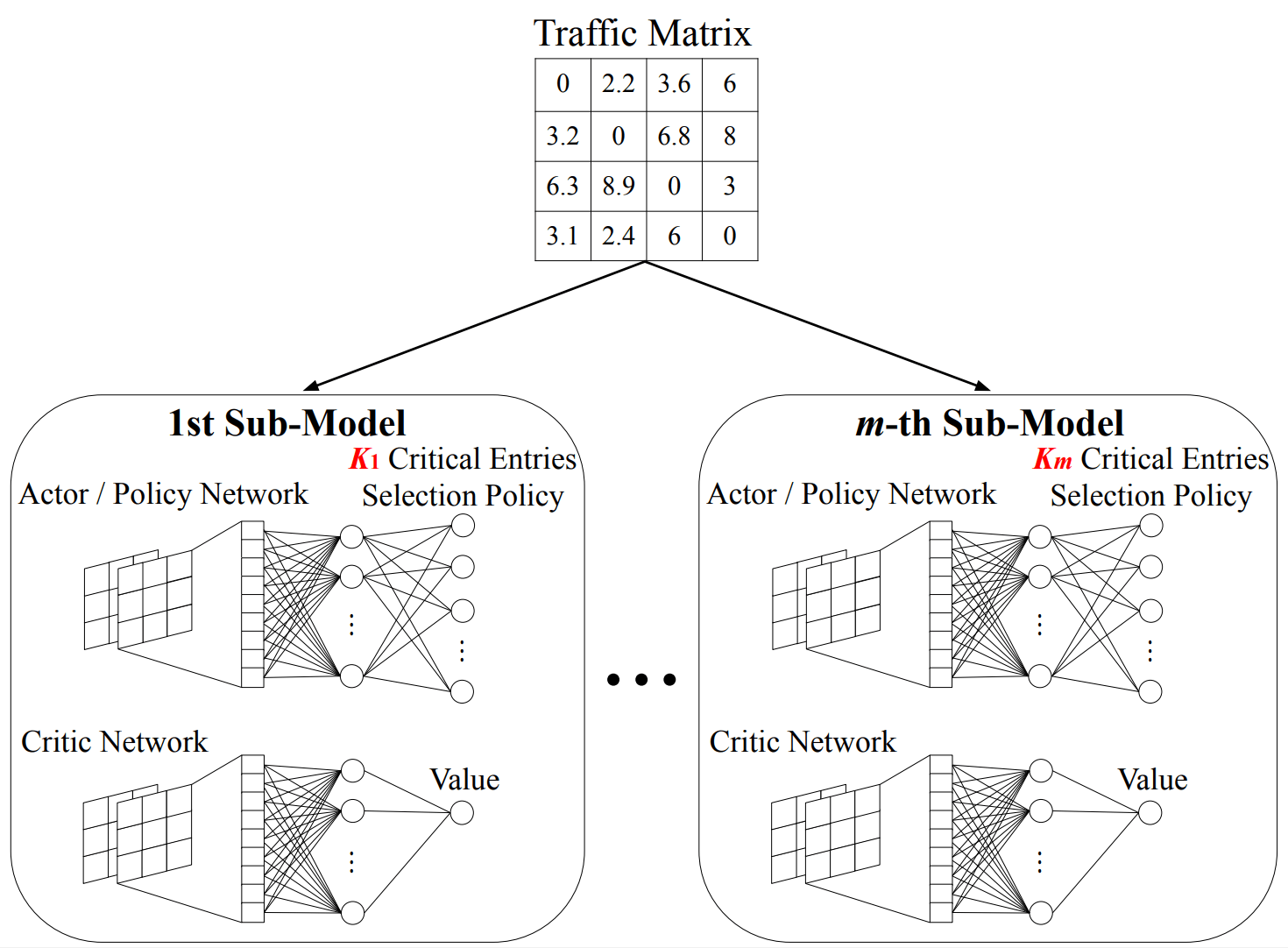
(4) Stage 2: Train a single RL model to learn a sub-model selection policy, such that FlexEntry can select flexible numbers of critical entries to accommodate dynamic traffic scenarios
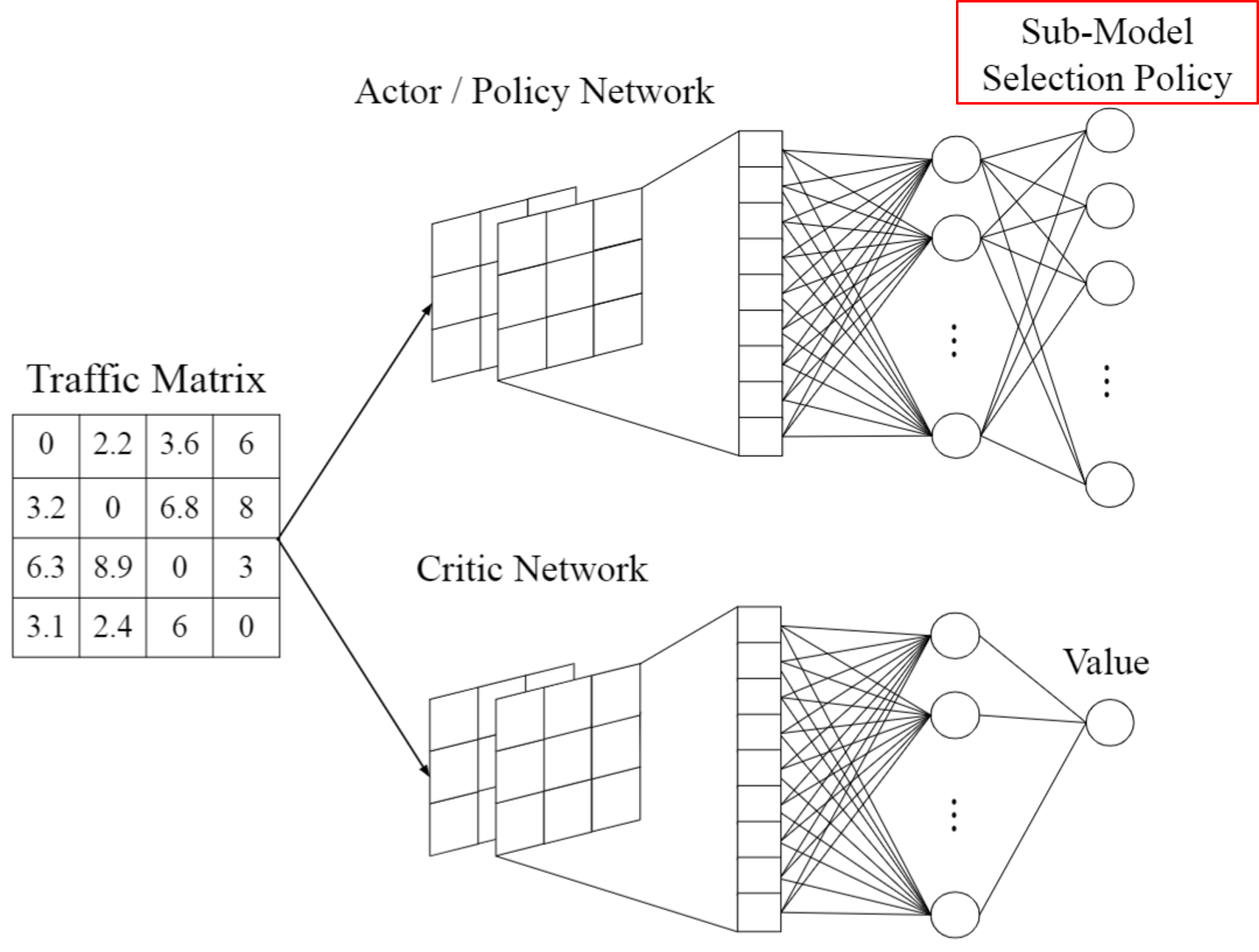
(5) Evaluation results: FlexEntry generalizes well to different traffic variations with near-optimal load balancing performance while only updating a very low percentage (~1%) of critical entries
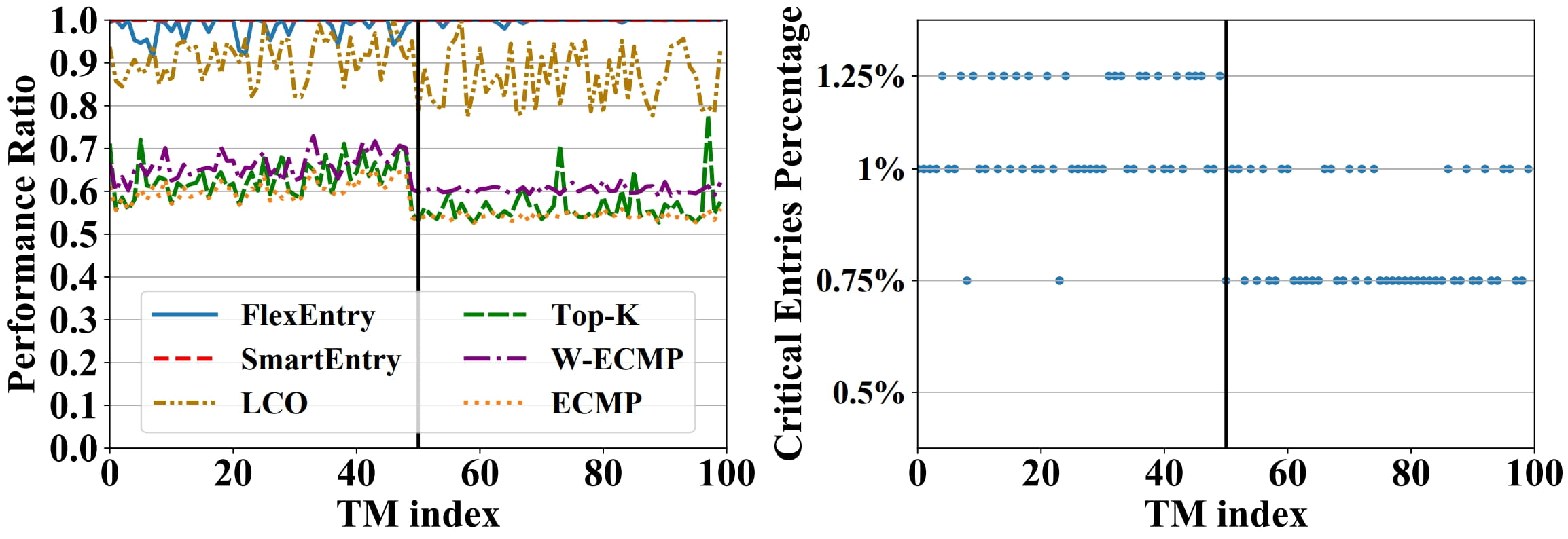
Contributions:
- We customized a 2-stage RL approach to identify critical destination-based forwarding entries for routing updates in different traffic scenarios.
- We adopted Linear Programming (LP) to produce reward signals for RL and optimize traffic split ratios for the selected critical entries to control traffic distribution.
- Our proposed TE solution achieved near-optimal performance in unseen traffic scenarios with at most 99.3% of average entry update savings in six real networks.
Abstract:
Traffic Engineering (TE) is a widely-adopted network operation to optimize network performance and resource utilization. Destination-based routing is supported by legacy routers and more readily deployed than flow-based routing, where the forwarding entries could be frequently updated by TE to accommodate traffic dynamics. However, as the network size grows, destination-based TE could render high time complexity when generating and updating many forwarding entries, which may limit the responsiveness of TE and degrade network performance.
In this paper, we propose a novel destination-based TE solution called FlexEntry, which leverages emerging Reinforcement Learning (RL) to reduce the time complexity and routing update overhead while achieving good network performance simultaneously. For each traffic matrix, FlexEntry only updates a few forwarding entries called critical entries for redistributing a small portion of the total traffic to improve network performance. These critical entries are intelligently selected by RL with traffic split ratios optimized by Linear Programming (LP).
We find out that the combination of RL and LP is very effective. Our simulation results on six real-world network topologies show that FlexEntry reduces up to 99.3% entry updates on average and generalizes well to unseen traffic matrices with near-optimal load balancing performance.
Publications:
- [JSAC 22] Minghao Ye, Yang Hu, Junjie Zhang, Zehua Guo, and H. Jonathan Chao, “Mitigating Routing Update Overhead for Traffic Engineering by Combining Destination-based Routing with Reinforcement Learning,” IEEE Journal on Selected Areas in Communications (JSAC), 2022. (Impact factor: 16.4) [Paper URL] [Codes] [PDF]
- [NetAI ’20] Junjie Zhang, Zehua Guo, Minghao Ye, and H. Jonathan Chao, “SmartEntry: Mitigating Routing Update Overhead with Reinforcement Learning for Traffic Engineering,” ACM SIGCOMM Workshop on Network Meets AI & ML (NetAI), 2020. (9 out of 19 papers were accepted) [Paper URL] [Slides] [Video] [PDF]